Jérémie Zumer successfully defends his doctoral thesis
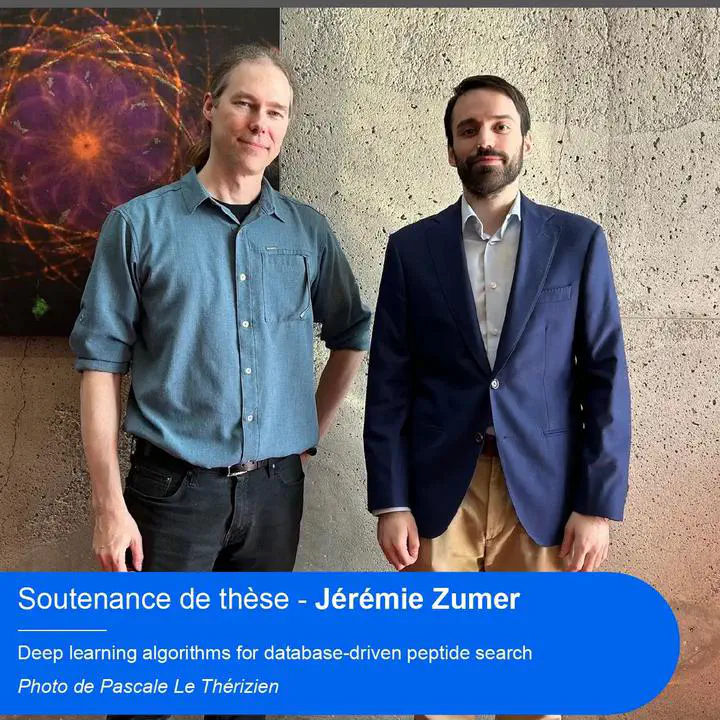
Congratulations to Dr. Jérémie Zumer for successfully completing his thesis defense! His thesis, entitled Deep learning algorithms for database-driven peptide search, will be available shortly in the Papyrus system of UdeM.
Modern proteomics – the large-scale analysis of proteins (Graves and Haystead, 2002) – relies heavily on the analysis of complex raw experimental, time series-like data. In a typical shotgun mass spectrometry workflow where the goal is to identify proteins in solution, a complex protein mixture is prepared, digested, fractionated for example by mass range, ionized and injected into a mass spectrometer, resulting in a so-called mass spectrum which, in tandem mass spectrometry, achieves obtain amino acid-resolution signals for the detected peptides. The spectrum must be cleaned up to become suitable for further analysis, then the peaks defined by the m/z to intensity values in the spectrum can be matched to some expected peak sequence from a set of candidate peptides (which are often simply in silico digests from the source specie’s proteome), which is the process of peptide identification proper. In this work, we select and solve some current limitations in the computational side of peptide identification research. We first introduce a new, research-oriented search engine. A major question at the boundary of current proteomics research is the integration and viability of new deep learning-driven algorithms for identification. Very little work has been done on this topic so far, with Prosit (Gessulat et al., 2019) being the only such software to see integration in an existing search engine, as far as we are aware (although rescoring algorithms like Percolator (Käll et al., 2007), which typically use more classical machine learning algorithms, have been in routine use for a while by now, they are merely applied as a postprocessing step and not integrated in the engine per se). To investigate this, we develop and present a new deep learning algorithm that performs peptide length prediction from a spectrum (a first, as far as we are aware). We compute metrics based on this prediction that we use during rescoring, and demonstrate consistently improved peptide identifications. Moreover, we propose a new full spectrum prediction algorithm (in line with PredFull (Liu et al., 2020) rather than Prosit) and a novel, random forest-based rescoring algorithm and paradigm, which we integrate within our search engine. Altogether, the deep learning tools we propose show an increase of over 20% in peptide identification rates at a 1% false discovery rate (FDR) threshold. These results provide strong evidence that deep learning-based tools proposed for proteomics can greatly improve peptide identifications.