Using deep learning diffusion models for denoising of low-coverage RNA-seq data
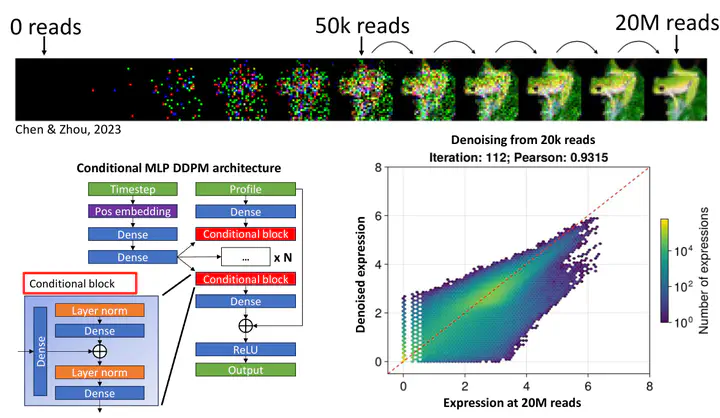
Although RNA sequencing (RNA-seq) allows us to gain deeper insight into human biology, the amount of samples that can be sequenced is still limited by sequencing costs. The cost per sample can be reduced by decreasing the sequencing depth, but this leads to lower quality data. Additionally, existing techniques to artificially increase data quality, such as imputation methods in single-cell RNA-seq, are not suitable for denoising standard RNA-seq data (bulk RNA-seq).
One model that has the potential to solve this problem is the diffusion model. We want to modify this model, originally created to iteratively generate synthetic images, by linking model iterations to sequencing depth. This would allow denoising RNA-seq data in such a way as to completely recover all biological information present at full coverage, such as sample type or differentially expressed genes.
This model has the potential to create new sequencing standards for RNA-seq in various experimental or hospital areas, making it possible to both reduce costs and provide large quantities of data.