Léonard Sauvé successfully defends his doctoral thesis
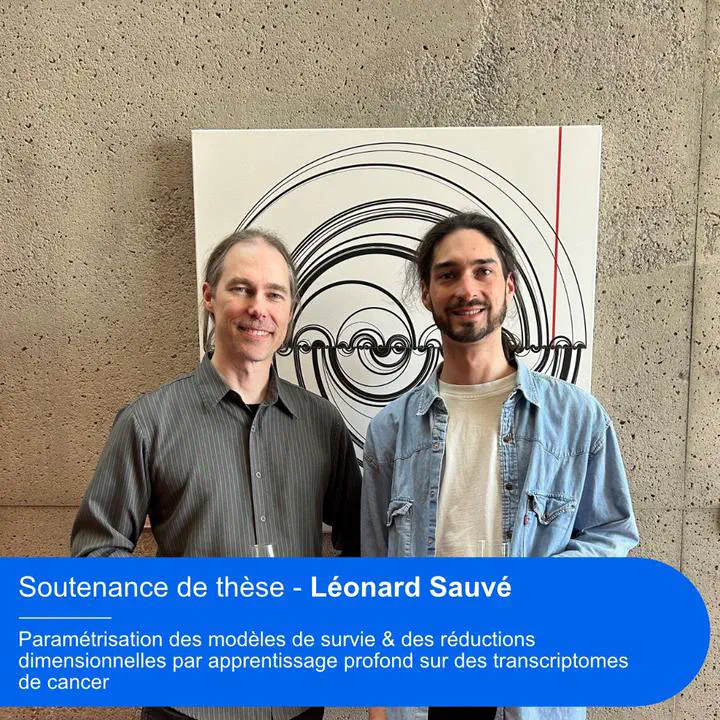
Congratulations to Dr. Léonard Sauvé for successfully defending his thesis! His thesis, entitled Parameterization of Survival Models & Dimensional Reduction by Deep Learning on Cancer Transcriptomes, is now available in the UdeM Papyrus system.
Survival analyses play an important role in cancer treatment research. Cancers carry numerous mutations and chromosomal rearrangements, while genetic and demographic variants describe individual-specific genetic factors. The acquisition of gene expression profiles of cancer through RNA sequencing allows the quantification of the direct effect of the individual’s and cancer’s genetic variants. The aim of this thesis is to measure the impact of dimensionality reduction for survival analyses, to develop a dimensionality reduction method for transcriptomic data, and to explore deep learning methods using dimensionality reductions that improve the performance of survival analyses. Two underlying objectives are intertwined with the work presented here: identifying the contributions of deep artificial neural networks to cancer survival prediction, if any, and determining the impact of hyperparameters necessary to obtain optimal models. Our results demonstrate how a published gene signature has a predictive ability for survival in AML with a standard survival model by concordance index equivalent to PCA, an informed dimensionality reduction method. We then show an adaptation to a published method necessary to achieve the convergence of a deep neural network. Finally, we describe the performance difference between the standard survival model and the model using this concordance index model. By using the entire profile, there doesn’t seem to be a difference, but informed low-dimensional reductions seem to favor deep networks. In the fourth chapter, the development of a dimensionality reduction method with Factorized Embeddings improved its computation time and demonstrated again that FE can be used as a 2D visualization technique. We also allowed trained FEs to infer new points and demonstrated that these can be used as a dimensionality reduction method for predicting an auxiliary task, and compared it to standard approaches. Additionally, we mapped certain links between the L2 hyperparameters and gene embedding size and the topology of the 2D embedding space of samples. Finally, we identified the current limitations of the model, guiding future research to improve the algorithm’s performance. The fifth chapter presents approaches that combine dimensionality reduction and deep learning to improve survival predictions. Two types of approaches are explored: one training a survival network and optimizing a dimensionality reduction network in a decoupled manner, and the other training both networks simultaneously. In the first case of decoupled learning, a dimensionality reduction network is trained first, then the deep survival network is trained on the data projection in the reduced space. In the second case, both parts of the network are trained simultaneously during optimization. In this section, we study two contrasting approaches: multitask learning using variational autoencoders and a deep Cox network, and another using transfer learning. Finally, it is analyzed that survival networks do not currently benefit from deep models. Their limited number of samples in the datasets and excessive dimensionality would be the main reasons. The dimensionality reductions explored in this thesis offer interesting improvement perspectives but deserve further exploration to provide significant improvements.