Caroline Labelle successfully defends her doctoral thesis
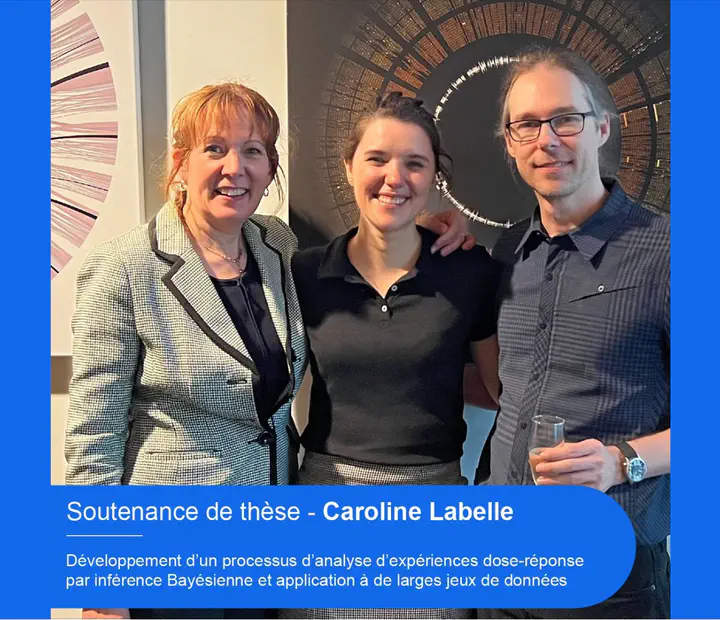
Congratulations to Dr. Caroline Labelle for successfully completing her thesis defense! Her thesis, entitled Development of an analyzing process for dose-response experiments by Bayesian inference and its application to large datasets, will be available shortly in the Papyrus system of UdeM.
In the context of drug discovery, various chemical compounds are developed, tested and optimized with the aim of identifying new effective therapies for a specific medical context. The efficiency of these compounds is characterized by efficiency metrics, such as potency and effi- cacy (HDR), that are derived from the analysis of dose-response experiments. Experimenters filter and select compounds based on these efficiency metrics. Traditionally, the values of efficiency metrics are estimated by adjusting the parameters of the log-logistic model to experimental data. I refer to this approach as Levenberg-Marquardt, the most commonly implemented algorithm for non-linear regression by gradient descent. Although Levenberg-Marquardt is the standard in the analysis of dose-response experiments, it presents the main limitation of not being able to adequately evaluate or quantify the uncertainty of efficiency metrics. This has a particularly harmful impact when incomplete or flat responses are analyzed: the estimated metrics are incorrect and experimenters are not equipped to quickly identify them. They often have to fall back on visual evaluations of responses, an approach that is not very effective when several experiments are considered and difficult to reproduce from one experimenter to another. There is thus a dire need for a robust and accessible methodology that account for uncertainty arising from the experimental data and is able to quantify the underlying uncertainty of efficiency metrics.
This thesis aims to provide experimenters with better tools for analyzing dose-response experiments and making decisions. To do this, I propose a Bayesian inference analysis process: the efficiency metrics are now represented by distributions of the most probable values, i.e. posteriors. The posteriors explicitly represent the uncertainty arising from biological, experimental and analytical variabilities. The integration of priors makes the inference process robust to incomplete or flat experiments, unlike Levenberg-Marquardt. I demonstrate this robustness qualitatively and quantitatively via a comparison of representations (i.e., posterior and estimation) for pairs of biological replicates from three large public datasets. Alongside the new proposed methodology, I demonstrate for the first time quantitatively the shortcomings of Levenberg-Marquardt. I also propose various post-inference analyzes drawing all the informative potential of the posteriors. Such analyzes are more flexible, informative and statistically valid than analyzes done on Levenberg-Marquardt estimates. Finally, I integrated the process of inference and post-inference analyzes into two versions of a web interface (BiDRA: Bayesian inference for the Analysis of Dose-Response) thus providing tools in a user-friendly manner for experimenters.
My thesis work proposes a robust and accessible alternative to the shortcomings of Levenberg-Marquardt in the context of characterizing the efficiency of chemical compounds. Additionally, the various demonstrations pave the way for the integration of Bayesian inference to the analysis various types of experiments in the context of the drug discovery process.