Léa Kaufmann rewarded for her poster at the 2024 Louis-Philippe-Bouthillier Meeting
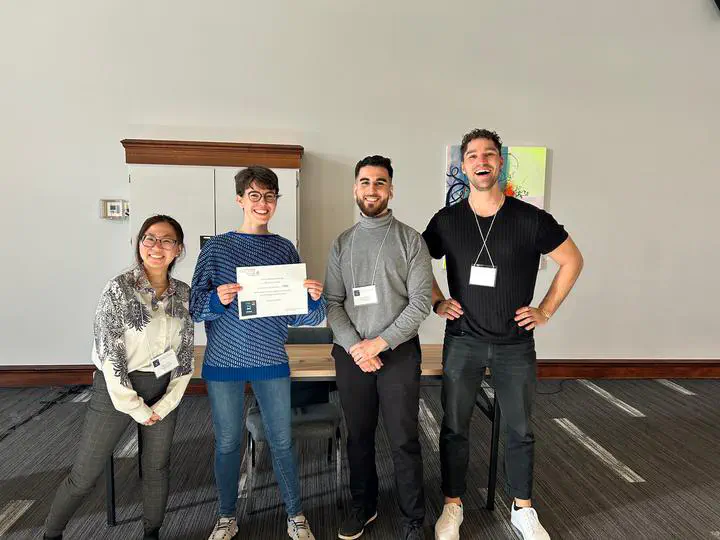
Congratulations to Léa Kaufmann who won the “People’s Choice” award for her poster presentation. The latter focused on the use of gene expression profiles for the prediction of biological activity of chemical compounds in cancer cells. Léa also had the opportunity to present her research work in the form of an oral presentation. Well done!
The Louis-Philippe-Bouthillier Meeting is organized by the Department of Biochemistry of the University of Montreal in honor of Professor Louis-Philippe Bouthillier. This meeting has now become an annual tradition which brings together all the members and research stakeholders of the department and thus allows everyone to benefit from this privileged forum to learn about and discuss the various research projects.
Use of gene expression profiles for the prediction of biological activity of chemical compounds in cancer cells
The analysis of compounds for the development of new drug therapies is a time-consuming and expensive process. Chemoinformatics aims to accelerate it through predictions based on the chemical structure of the compound, but it does not take biological interactions into account. Our hypothesis is that the transcriptomic profile of a cell treated with a chemical compound is a better representation of the compound than the structure of the latter for the prediction of its activity. As primary cells are often available in very small quantities and have a limited lifespan outside the human body, a large number of compounds cannot be screened on them. The goal of this project is to be able to predict the effect of untested chemical compounds on a patient’s cells in order to accelerate the choice of a suitable treatment. We use the LINCS dataset from the Broad Institute which contains more than 3 million transcriptomic profiles and the ChemBank dataset (Petri Seiler et al., 2008) which contains 2,500 biological assays from high-throughput screening. We seek to predict test results from transcriptomic profiles using a Deep Neural Network model. We have previously shown the feasibility of predicting a gene of a LINCS cell line from the transcriptomic profiles of another lineage. Our work will make it possible to identify in silico compounds that would be good candidates for the pre-clinical phase of drug development against certain cancers. expensive. Chemoinformatics aims to accelerate it through predictions based on the chemical structure of the compound, but it does not take biological interactions into account.