Bayesian Inference as a Robust Alternative to Non-Linear Regression for Dose-Response Parameters Assessment at ISMB 2022
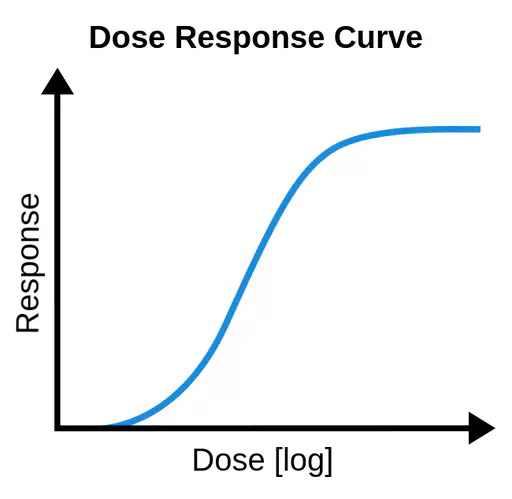
Caroline Labelle’s presentation: Bayesian Inference as a Robust Alternative to Non-Linear Regression for Dose-Response Parameters Assessment, has been accepted at the 2022 edition of the International Conference on Intelligent Systems for Molecular Biology (ISMB). The poster abstract can be found online.
Abstract
Large-scale dose-response screens are used to test efficacy of therapeutic agents for various conditions. We previously proposed a Bayesian inference model (BiDRA) for the assessment of dose-response parameters.
Using large-scale pharmacological datasets, we demonstrate the robustness and the gain of using BiDRA, compared to the standard Levenberg-Marquardt algorithm. Notably, we demonstrate that discrepancies in replicated experiments are in part due to the analytical approach of obtaining dose-response parameters.
We identify the main limitation of Levenberg-Marquardt as being incomplete and seemingly unresponsive responses curves. For such experiments, Levenberg-Marquardt either do not converge (for a given number of iterations) or forces a fit and return unreliable parameters. We identify those experiments as being an important driver of discrepancies. For instance, for replicates of incomplete responses curves, Levenberg-Marquardt’s HDR (high-dose response) estimates are arbitrary and often discordant, even though the experiments are concordant in the sense that their HDRs are unobservable. Alternatively, BiDRA’s posterior distributions are representative of the uncertainty of the parameters and align with one another, suggesting that the experiments are indeed concordant. Overall, we observe that BiDRA’s posterior distributions have higher correlation coefficients when comparing the parameters of replicated experiments.